Harnessing AI: Innovative Solutions for Climate Change
- April 17, 2024
- Posted by: Cheryl Li
- Category: AI & AI Automation Trends
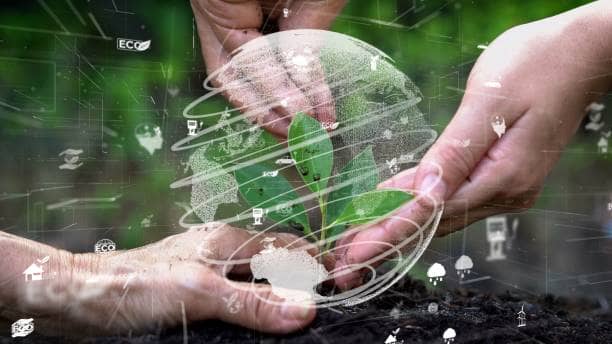
Climate change represents one of the most pressing challenges of our time. Rising global temperatures, extreme weather events, and the depletion of natural resources are just a few of the consequences we face. As the world grapples with these issues, technology has emerged as a beacon of hope. Artificial Intelligence (AI), in particular, holds immense potential in addressing climate change by offering innovative solutions to mitigate its impact and adapt to its consequences. However, the deployment of AI in this context is not without its challenges. This blog post explores the role of AI in combating climate change, highlighting both the solutions it offers and the challenges it faces.
AI-Driven Solutions for Climate Change
Predictive Modeling and Climate Forecasting
AI excels in analyzing vast amounts of data, making it a powerful tool for predictive modeling and climate forecasting. Machine learning algorithms can process data from satellites, weather stations, and historical climate records to predict future climate patterns and extreme weather events with greater accuracy.
Early Warning Systems
AI-powered early warning systems can predict natural disasters such as hurricanes, floods, and wildfires. These systems analyze meteorological data to provide timely alerts, enabling communities to prepare and respond more effectively. Early warnings can save lives, reduce economic losses, and enhance resilience to climate impacts.
Climate Scenario Analysis
AI can simulate various climate scenarios based on different levels of greenhouse gas emissions and policy interventions. These simulations help policymakers understand the potential outcomes of their decisions and develop strategies to mitigate climate change.
Renewable Energy Optimization
The transition to renewable energy sources is crucial for reducing greenhouse gas emissions. AI plays a significant role in optimizing the production and distribution of renewable energy.
Smart Grids
AI-powered smart grids enhance the efficiency of electricity distribution by analyzing consumption patterns and adjusting supply accordingly. This reduces energy waste and ensures a stable and reliable power supply. Smart grids also facilitate the integration of renewable energy sources like solar and wind into the energy mix.
Predictive Maintenance
AI can predict when renewable energy equipment, such as wind turbines and solar panels, will need maintenance or replacement. Predictive maintenance reduces downtime and ensures that renewable energy systems operate at peak efficiency.
Energy Storage Management
AI algorithms optimize the use of energy storage systems, such as batteries, by predicting energy demand and supply fluctuations. This ensures that excess energy generated during peak production periods is stored and used when demand is high, balancing the grid and reducing reliance on fossil fuels.
Sustainable Agriculture
Agriculture is both a contributor to and a victim of climate change. AI offers solutions to make agriculture more sustainable and resilient.
Precision Farming
AI-driven precision farming techniques use data from sensors, drones, and satellite imagery to monitor crop health, soil conditions, and weather patterns. This information allows farmers to make data-driven decisions about irrigation, fertilization, and pest control, reducing resource use and improving crop yields.
Climate-Resilient Crops
AI can analyze genetic data to identify traits that make crops more resilient to climate change. By accelerating the development of climate-resilient crop varieties, AI helps ensure food security in the face of changing weather patterns.
Carbon Sequestration
AI is instrumental in identifying and enhancing carbon sequestration methods, which are critical for reducing atmospheric CO2 levels.
Forest Management
AI can monitor forest health and track deforestation in real time using satellite imagery and remote sensing data. This helps in the implementation of conservation strategies and reforestation efforts to enhance carbon sequestration.
Soil Carbon Sequestration
AI models can predict the carbon sequestration potential of different soil management practices. By recommending optimal practices, AI helps increase the amount of carbon stored in soil, contributing to overall emissions reduction.
Challenges in Leveraging AI for Climate Change
Data Availability and Quality
AI’s effectiveness depends on the availability and quality of data. In many parts of the world, especially in developing countries, there is a lack of reliable and comprehensive climate data. This hampers the development of accurate predictive models and limits the effectiveness of AI-driven solutions.
Data Gaps
Significant data gaps exist in regions with limited monitoring infrastructure. Bridging these gaps requires substantial investment in data collection technologies and international collaboration to share data across borders.
Data Privacy and Security
Collecting and sharing climate data raise concerns about privacy and security. Ensuring that data is used responsibly and protecting it from misuse is essential for maintaining public trust and cooperation.
Computational Resources
AI applications, particularly those involving deep learning, require significant computational resources. The energy consumption associated with running large-scale AI models can be substantial, potentially offsetting some of the environmental benefits they offer.
Energy-Intensive Training
Training AI models, especially in climate simulations and predictive analytics, demands high computational power. Finding ways to reduce the energy consumption of these processes, such as using more efficient algorithms and renewable energy sources, is crucial.
Access to Technology
Developing countries often lack access to advanced computational resources, limiting their ability to leverage AI for climate change mitigation and adaptation. Ensuring equitable access to technology and computational infrastructure is necessary for global climate action.
Ethical and Social Considerations
The deployment of AI in climate action raises ethical and social considerations that must be addressed to ensure fair and equitable outcomes.
Bias and Fairness
AI models can inadvertently perpetuate biases present in the training data, leading to unfair outcomes. Ensuring that AI systems are designed and implemented with fairness in mind is essential for their effectiveness and acceptance.
Socio-Economic Impacts
The transition to AI-driven solutions may have socio-economic impacts, such as job displacement in certain industries. Addressing these impacts through policies that support workforce transition and skills development is necessary for a just and inclusive approach to climate action.
Integration and Scalability
Integrating AI solutions into existing systems and scaling them up to have a meaningful impact on climate change is a complex challenge.
Interoperability
Ensuring that AI systems can communicate and work seamlessly with existing technologies and infrastructure is crucial for their successful implementation. This requires standardization and collaboration across industries and sectors.
Scaling Solutions
Many AI-driven climate solutions are currently at the pilot stage. Scaling these solutions to have a global impact requires significant investment, coordination, and political will.
Conclusion
AI offers transformative potential in the fight against climate change, providing innovative solutions for predictive modeling, renewable energy optimization, sustainable agriculture, and carbon sequestration. However, realizing this potential requires overcoming challenges related to data availability, computational resources, ethical considerations, and scalability. By addressing these challenges through international collaboration, investment in technology, and inclusive policies, we can harness the power of AI to create a sustainable and resilient future. The fight against climate change is a collective effort, and AI stands as a powerful ally in this critical endeavor.