The Importance of Data Quality in AI: Ensuring Reliable and Effective Outcomes
- November 9, 2024
- Posted by: Cheryl Li
- Category: AI Technology Deep Dives
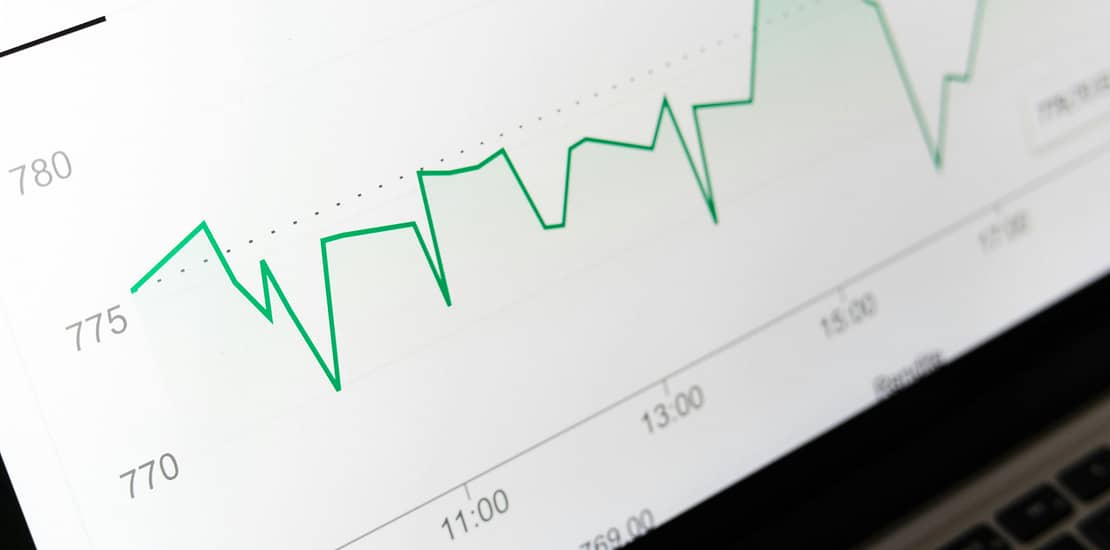
In the rapidly advancing field of artificial intelligence (AI), data is often referred to as the new oil. This comparison highlights the critical role that data plays in powering AI systems. However, just as oil must be refined to be useful, data must also meet certain standards of quality to be truly valuable for AI applications. The importance of data quality in AI cannot be overstated; it directly impacts the accuracy, reliability, and effectiveness of AI models. This blog post delves into why data quality is essential in AI, the dimensions of data quality, the challenges in maintaining high data quality, and best practices for ensuring data quality in AI projects.
Why Data Quality Matters in AI
Impact on Model Accuracy
The accuracy of AI models hinges on the quality of the data they are trained on. High-quality data enables models to learn accurate patterns and make precise predictions. Conversely, poor-quality data can lead to models that produce unreliable or incorrect outcomes. In fields such as healthcare, finance, and autonomous driving, the consequences of inaccurate models can be severe, underscoring the need for robust data quality.
Model Generalization
AI models are designed to generalize from the data they are trained on to new, unseen data. High-quality data that is representative of the real-world scenarios ensures that models perform well in varied conditions. Data that is incomplete, biased, or noisy can impair a model’s ability to generalize, resulting in poor performance when deployed in real-world applications.
Ethical and Fair AI
Data quality is also a cornerstone of ethical and fair AI. Biased or unrepresentative data can perpetuate existing inequalities and lead to biased AI systems. Ensuring data quality involves checking for and mitigating biases in the data, thereby contributing to the development of fair and ethical AI systems that do not discriminate against any group.
Cost Efficiency
Investing in high-quality data from the outset can save significant costs in the long run. Poor-quality data can lead to the development of flawed models, requiring rework, additional testing, and retraining, all of which consume time and resources. High-quality data reduces the need for these costly iterations.
Dimensions of Data Quality
Data quality is multi-faceted and can be assessed across several dimensions:
Accuracy
Accuracy refers to how close data values are to the true values. Accurate data ensures that AI models learn correct patterns and make reliable predictions.
Completeness
Completeness indicates whether all required data is present. Incomplete data can lead to gaps in model learning and potentially incorrect conclusions.
Consistency
Consistency means that data is uniform and consistent across different datasets and sources. Inconsistencies can confuse AI models and degrade their performance.
Validity
Validity checks whether the data conforms to predefined formats and ranges. Invalid data can lead to errors in data processing and model training.
Timeliness
Timeliness refers to the relevance of data at the time it is used. Outdated data can mislead models, especially in rapidly changing environments.
Uniqueness
Uniqueness ensures that there are no duplicate records in the dataset. Duplicates can skew model learning and lead to biased outcomes.
Challenges in Ensuring Data Quality
Data Collection
The first challenge in ensuring data quality arises during data collection. Data can come from various sources, including sensors, databases, and user inputs, each with its own potential for inaccuracies and inconsistencies. Ensuring quality at the collection stage requires robust processes and technologies to filter and verify data.
Data Integration
Combining data from multiple sources can introduce inconsistencies and redundancies. Harmonizing data to ensure consistency and completeness while preserving accuracy is a complex task that requires meticulous planning and execution.
Data Labeling
In supervised learning, data labeling is crucial as it provides the ground truth for model training. Inaccurate or inconsistent labeling can significantly degrade model performance. Ensuring high-quality labeling involves clear guidelines, rigorous training for annotators, and thorough validation processes.
Data Preprocessing
Data preprocessing steps such as cleaning, normalization, and transformation are essential to prepare raw data for AI models. Errors in preprocessing can introduce biases or inaccuracies that compromise data quality. Automated preprocessing tools, coupled with human oversight, can help maintain high standards.
Dynamic and Evolving Data
In many applications, data is not static but evolves over time. Keeping the data up-to-date and relevant requires continuous monitoring and updating processes. This dynamic nature of data poses ongoing challenges to maintaining data quality.
Best Practices for Ensuring Data Quality in AI
Implement Robust Data Governance
Data governance involves setting policies and standards for data management across the organization. A robust data governance framework ensures accountability, defines data quality metrics, and establishes processes for maintaining data quality. This includes regular audits and compliance checks.
Automated Data Quality Tools
Leveraging automated tools for data quality assessment can significantly enhance the efficiency and effectiveness of quality control processes. These tools can automatically detect anomalies, inconsistencies, and missing values, facilitating timely interventions.
Data Quality Metrics and Monitoring
Defining clear data quality metrics and continuously monitoring these metrics is crucial. Metrics such as accuracy, completeness, and consistency should be tracked over time, with deviations promptly addressed. Automated dashboards and alerts can help in real-time monitoring and issue resolution.
Regular Data Cleaning
Regular data cleaning is essential to remove inaccuracies, duplicates, and irrelevant data. This involves standardized procedures for data validation, correction, and deduplication. Automated cleaning tools can assist in maintaining data quality at scale.
Training and Documentation
Investing in training for data handlers and maintaining comprehensive documentation are critical for ensuring data quality. Clear documentation of data sources, collection methods, preprocessing steps, and labeling guidelines helps maintain consistency and accuracy across the data lifecycle.
Ethical Considerations and Bias Mitigation
Proactively addressing ethical considerations and bias in data is vital for developing fair AI systems. This involves diverse and representative data collection, bias detection tools, and inclusive data governance practices. Regular bias audits and stakeholder consultations can help mitigate potential biases.
Continuous Improvement
Data quality management should be an ongoing effort. Continuous improvement processes, including feedback loops from model performance, user feedback, and data audits, help identify and address quality issues proactively. Adopting a culture of continuous improvement ensures long-term data quality.
Conclusion
Data quality is the foundation upon which effective and reliable AI systems are built. High-quality data ensures model accuracy, enhances generalization, supports ethical AI, and contributes to cost efficiency. However, maintaining data quality is a multifaceted challenge that requires robust governance, automated tools, clear metrics, regular cleaning, thorough training, and a commitment to continuous improvement. By prioritizing data quality, organizations can unlock the full potential of AI, driving innovation and achieving better outcomes in various domains. In the rapidly evolving landscape of AI, data quality will remain a critical determinant of success.