Sustainable AI: Navigating the Environmental Impact of Artificial Intelligence
- March 8, 2024
- Posted by: Cheryl Li
- Category: AI Ethics & Governance
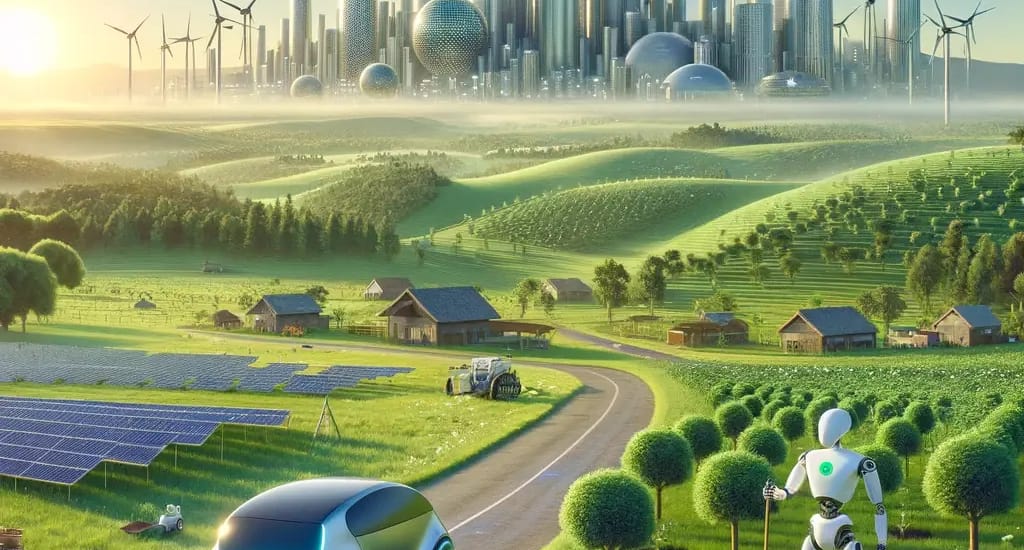
In the age of rapid technological advancement, Artificial Intelligence (AI) stands out as a beacon of innovation, driving changes across industries from healthcare to transportation. However, the environmental footprint of developing, deploying, and maintaining AI systems has sparked a crucial conversation about sustainable AI. This blog post delves into the environmental considerations of AI, exploring the challenges it poses, the strategies for mitigating its impact, and the role of AI in promoting sustainability.
The Environmental Footprint of AI
The environmental impact of AI is multifaceted, stemming from the energy-intensive nature of training complex AI models, the hardware required to support these systems, and the lifecycle of the devices and infrastructure that underpin AI technologies.
Energy Consumption in AI Training and Operations
Training sophisticated AI models, particularly in fields like deep learning, requires substantial computational power, leading to significant energy use. Data centers, which house the servers running these AI models, consume vast amounts of electricity, contributing to carbon emissions and environmental degradation.
Hardware Production and Waste
The rapid pace of AI development necessitates frequent upgrades of hardware components like GPUs and specialized AI processors. This cycle of production and disposal contributes to electronic waste (e-waste), further exacerbating the environmental impact.
Challenges in Achieving Sustainable AI
The path to sustainable AI is fraught with challenges, including the increasing complexity of AI models, the demand for real-time AI applications, and the global nature of AI development and deployment.
Scaling Complexity of AI Models
As AI models become more sophisticated, their energy requirements escalate. Models like GPT-3, with billions of parameters, exemplify this trend, raising concerns about the sustainability of pursuing ever-larger AI architectures.
Real-time Applications and Continuous Operations
AI applications that require continuous operation, such as autonomous vehicles and real-time monitoring systems, consume energy around the clock, highlighting the need for sustainable energy sources to support these technologies.
Global Disparities in Energy Sources
The environmental impact of AI varies significantly across regions, depending on local energy sources. Data centers powered by renewable energy have a lower environmental footprint compared to those relying on fossil fuels, underscoring the importance of clean energy in sustainable AI.
Strategies for Mitigating the Environmental Impact of AI
Addressing the environmental challenges of AI necessitates a multifaceted approach, combining technological innovations with policy interventions and industry-wide commitments to sustainability.
Efficient AI Algorithms and Architectures
Developing more efficient AI algorithms and architectures can reduce the computational power—and consequently, the energy—required to train and run AI models. Techniques like model pruning, quantization, and knowledge distillation can streamline AI models without compromising performance.
Adoption of Green Computing Practices
Green computing practices, such as optimizing data center operations, utilizing energy-efficient hardware, and adopting renewable energy sources, can significantly reduce the carbon footprint of AI systems.
Lifecycle Management of AI Hardware
Implementing robust recycling and reuse programs for AI hardware can mitigate the environmental impact of electronic waste. Additionally, designing hardware with longer lifecycles and upgradability can minimize the need for frequent replacements.
Cross-sector Collaborations and Policies
Collaboration across the tech industry, academia, and governments is crucial in promoting sustainable AI. Policies and regulations that encourage or mandate the use of renewable energy, energy-efficient technologies, and sustainable practices can drive systemic change.
The Role of AI in Promoting Environmental Sustainability
While AI poses environmental challenges, it also holds immense potential to address sustainability issues. AI can optimize energy use, reduce waste, and enhance the efficiency of renewable energy sources, contributing to a more sustainable future.
AI in Energy Optimization
AI can optimize energy consumption in various sectors, from smart grids that balance electricity supply and demand to AI-driven building management systems that reduce energy use.
AI for Environmental Monitoring and Protection
AI applications in environmental monitoring can track deforestation, ocean health, and air quality, providing valuable data to inform conservation efforts and policy-making.
Enhancing the Efficiency of Renewable Energy
AI can improve the efficiency and reliability of renewable energy sources, such as predicting wind patterns for wind farms or optimizing the operation of solar panels, making renewable energy more viable and accessible.
Navigating the Future of Sustainable AI
The journey towards sustainable AI is ongoing, requiring continued innovation, awareness, and commitment to environmental stewardship. As AI technologies evolve, so too must our approaches to minimizing their environmental impact while leveraging AI to tackle pressing sustainability challenges.
Continuous Innovation for Sustainability
The tech community must prioritize research and development in sustainable AI, exploring new methods, technologies, and materials that reduce the environmental footprint of AI systems.
Global Standards and Best Practices
Establishing global standards and best practices for sustainable AI can provide a framework for the industry, guiding companies in integrating environmental considerations into their AI initiatives.
Leveraging AI for Sustainability Goals
AI should be harnessed not just for economic and social benefits but also as a tool for achieving sustainability goals, from climate change mitigation to resource conservation.
Conclusion
The intersection of AI and environmental sustainability is a complex but critical domain, demanding thoughtful consideration and action. By acknowledging the environmental implications of AI and adopting strategies to mitigate its impact, we can steer the development of AI towards a path that is not only technologically advanced but also environmentally responsible. In doing so, we harness the full potential of AI as a catalyst for positive change, contributing to a sustainable future where technology and ecology harmoniously coexist.