Navigating the Maze: Overcoming Challenges in AI Adoption
- October 13, 2023
- Posted by: Cheryl Li
- Category: AI Strategy & Implementation
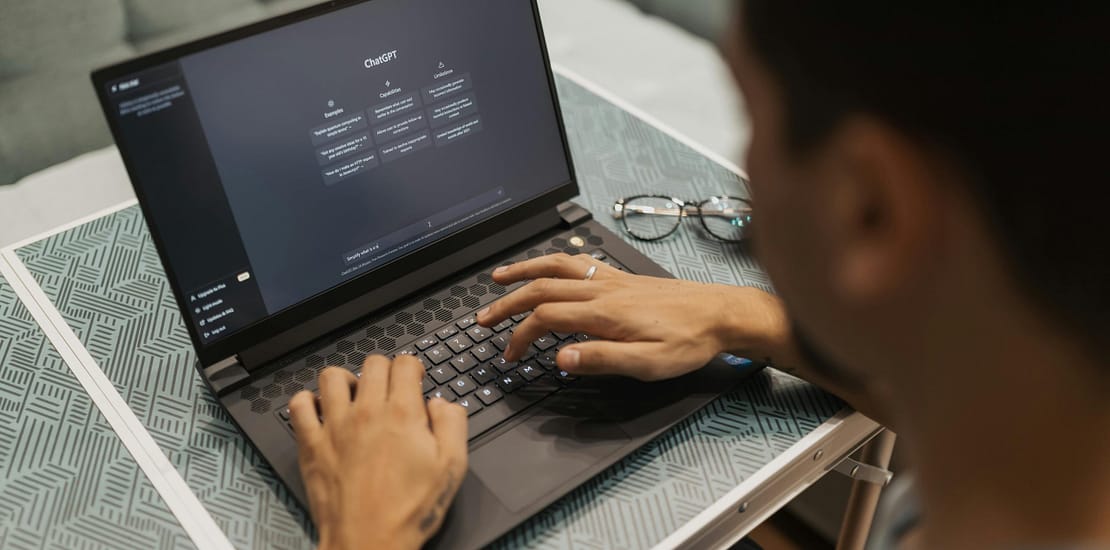
The adoption of Artificial Intelligence (AI) across various sectors has been transformative, offering unprecedented opportunities for innovation, efficiency, and competitive advantage. However, the journey towards AI integration is fraught with challenges that can hinder its successful implementation. From technical hurdles to ethical dilemmas, businesses embarking on the AI journey must navigate a complex landscape. This blog post explores the common challenges in AI adoption and provides strategies for overcoming them, ensuring a smoother path to harnessing AI’s full potential.
Deciphering the Complexity of AI
AI’s inherent complexity is one of the first obstacles businesses encounter. Understanding AI technologies, algorithms, and their applications can be daunting, especially for organizations new to the digital transformation arena. Demystifying AI through education and training is crucial. Businesses should invest in workshops, seminars, and courses for their teams, focusing on the basics of AI and its relevance to their specific industry. This foundational knowledge empowers teams to make informed decisions about AI projects and their implementation.
Aligning AI with Business Strategy
Integrating AI without a clear alignment to business objectives can lead to misguided efforts and wasted resources. The challenge lies in identifying where AI can add the most value. To overcome this, businesses should conduct thorough analyses to pinpoint processes or areas that could benefit from automation, enhanced decision-making, or predictive analytics. Setting clear, measurable goals for AI initiatives ensures that they contribute to overarching business objectives, providing direction and purpose to AI adoption efforts.
Tackling Data-Related Issues
Data is the lifeblood of AI. Yet, issues related to data quality, quantity, and governance can significantly impede AI projects. Many organizations struggle with siloed data, inconsistent formats, or incomplete datasets, making it difficult to train AI models effectively. Addressing these challenges requires a robust data management strategy, focusing on data integration, cleansing, and enrichment processes. Additionally, implementing strict data governance policies ensures data quality and compliance with regulatory standards, laying a solid foundation for AI initiatives.
Bridging the Talent Gap
The demand for AI expertise often outstrips supply, leaving businesses scrambling to find or develop talent. Overcoming this challenge involves a two-pronged approach: talent acquisition and internal talent development. Organizations should look to recruit specialists with AI experience while also providing training programs to upskill existing employees. Creating a culture of continuous learning and innovation encourages teams to develop the skills necessary for AI adoption and integration.
Ensuring Ethical AI Use
As AI spreads more widely, the importance of ethical considerations grows.Issues of bias, privacy, and accountability pose significant challenges. To navigate these, businesses must adopt ethical AI frameworks that guide the development and deployment of AI systems. These frameworks should emphasize transparency, fairness, and respect for user privacy, ensuring AI solutions are developed responsibly. Engaging with stakeholders, including customers and employees, about AI’s ethical implications fosters trust and transparency.
Overcoming Technical Integration Challenges
Integrating AI into existing IT infrastructures can be fraught with technical challenges, from compatibility issues to scalability concerns. A thorough assessment of the current IT landscape is essential, identifying potential integration points and bottlenecks. Leveraging cloud-based AI solutions can offer flexibility and scalability, easing integration woes. Additionally, adopting agile development methodologies allows for iterative testing and integration, minimizing disruptions to existing systems.
Managing Change and Cultural Resistance
The introduction of AI can be met with resistance from employees, driven by fears of job displacement or mistrust of automated systems. Managing this cultural shift is vital for successful AI adoption. Transparent communication about AI’s role and its benefits can alleviate fears. Involving employees in the AI implementation process, through training or participation in pilot projects, fosters a sense of ownership and acceptance, easing the transition to AI-enhanced workflows.
Navigating Regulatory and Compliance Issues
Regulatory and compliance concerns, particularly in industries like healthcare and finance, can complicate AI adoption. Staying abreast of relevant regulations and ensuring AI systems comply is crucial. Collaborating with legal and compliance teams during the development and deployment of AI solutions ensures that regulatory requirements are met, preventing potential legal issues down the line.
Sustaining AI Initiatives
Sustaining momentum in AI initiatives can be challenging, especially when initial excitement wanes or projects face setbacks. Continuous monitoring, evaluation, and adaptation of AI projects are essential. Setting up a governance structure for AI initiatives, with clear roles, responsibilities, and oversight, ensures they remain aligned with business goals and continue to deliver value.
Conclusion
While the path to AI adoption is strewn with challenges, they are not insurmountable. By understanding and strategically addressing these hurdles, businesses can pave the way for successful AI integration. Education, strategic alignment, robust data management, ethical considerations, and ongoing engagement are key to navigating the AI adoption maze. As organizations become more adept at overcoming these challenges, the potential of AI to transform businesses and industries becomes increasingly attainable, unlocking new horizons of innovation and growth.